Noise (human judgment)
{{AFC comment|1=The draft reads too much like an essay. The text has to be converted into prose format rather than bullet points, and avoid naming sections with over-extended descriptions, like "What noise is and its relationship to bias." Wingwatchers (talk) 04:21, 30 December 2021 (UTC)}
Script error: No such module "Draft topics".
Script error: No such module "AfC topic".
Noise in human judgment is "undesirable variability in judgments of the same problem".[1](pp37–38) It is a concept associated with fields such as psychology, judgment, decision-making science, behavioral science and statistics. Noise can lead to unfairness and to other problematic treatment of people in domains such as court cases, forensics, medicine, staff recruitment, performance evaluation and business strategy.[2]
There are several different techniques and procedures that can reduce noise, such as debiasing, use of algorithms/rules, use of guidelines, use of relative scales, use of base rates, aggregation of judgments and structured and carefully sequenced decision-making processes.[1] One common critique against certain types of noise reduction is that such efforts dehumanize people by taking away judges' agency.[3] Another common critique against certain types of noise reduction is that noise reduction techniques can in themselves increase or entrench discrimination and other types of bias against certain groups, such as racial bias.[4][5]
What noise is and its relationship to bias[edit]
When and why noise arises[edit]
Noise in human judgment comes in several forms:[1]
- Judgments are noisy when different judges are in disagreement with each other when assessing the same problem/case. (By 'judge' is generally meant anyone making a judgment, not only judges in the judiciary.)
- Judgments are noisy if a judge is in disagreement with her-/himself.
- A judgment made only once and only by one person/organization/group can also be noisy, since the judgment can be viewed as only one possible outcome in a cloud of possible judgments that the judge in question could have arrived at. However, note that it is not possible to measure noice in singular decisions, since there is only one data point and therefore no variability.
The reasons for why noise arises include the following:[1]
- Cognitive biases affecting judgments. Many documented cognitive biases can produce noise. Examples are prejudice, availability bias, irrationally long-lasting effects of first impressions, overconfidence, and confirmation bias.
- Differences in skill among judges, either in the judgment as a whole or in sub-parts of it.
- Differences in "taste" (preferences) between judges, for instance that a judge cares more about certain things than others.
- Judges' personal histories and emotional reactions. For instance, if something in the case/problem resonates with the judge on a personal level, that can affect their judgment in a way that would not be the case for another judge looking at the same case/problem.
- Mood and other transient factors such as energy level, fatigue and stress.
- Group dynamics. Group decision making and social influence often amplifies noise due to various factors influencing how judges deliberate, including because these group dynamics can lead to a lack of independence among judgments.
Relationship between noise and bias[edit]
Noise and how noise relates to bias in human judgment has been illustrated by a shooting range analogy[2], as shown in Fig 1, which shows what noise and statistical bias, respectively, each mean for error in judgment. An important distinction is that between statistical bias and psychological/cognitive bias. The latter can affect both statistical bias and noise.

- Target a): The bullseye represents full accuracy in the judgment. Since all shots here are in or close to the bullseye, the judgments are accurate. There is no bias on average and little noise.
- Target b): Compared to target a), there is less accuracy here. Instead, there is bias, because the shots are systematically off in one direction, down to the right from the bullseye. Put differently, there is an error on average (bias has to do with the mean value). However, since the shots are bundled close together they are precise; there is little noise.
- Target c): Here there is no error on average, because the errors due to the imprecision in each shot in relation to the bullseye cancel each other out. So there is no bias. However, there is much noise, because the shots differ much from each other. The standard deviation – a unit for measuring noise – is large. The individual or team making these shots is noisy.
- Target d): The individual or team making these shots has it worst, being both biased and noisy. Therefore, their error is the largest and they will have the steepest challenge if they attempt to become more accurate.
If there were no targets, just a canvas, it would be impossible to say whether any of the sets of shots are biased. This is because there would be no true value (bullseye) to compare the shots to. However, it would still be obvious that the c) and d) shots are noisy. Moreover, the illustration provides another way to understand that there is noise even in singular decisions: if only the first shot was observed, it would not be possible to know how much noise there will end up being, but the sources of noise are nevertheless already present in the shooter/shooters making the next shots.[1]
Examples of noisy judgments[edit]
Detecting noise is easiest when decisions made by many different judges are looked at simultaneously.[1] This has been done in many studies in different domains:
- Fairness: a study[6] on 208 criminal judges showed that their independently given punitive recommendations on 16 fictive cases varied greatly in harshness. For example, the judges only unanimously recommended imprisonment in three of the cases, and while the recommended number of prison years in one case was 1.1 years on average, one recommendation was as high as 15 years. In education, a study[7] looked at 682 real decisions by college admissions officers and found that the officers awarded the academic strengths of applicants more importance on cloudy days and, conversely, favored nonacademic strengths on sunny days. Moreover, French court judges have been found to be more lenient if it happens to be the defendant’s birthday.[8]
- Health and safety: one study[9] showed that whereas some radiologists never produced false negatives (missed real breast cancer) when examining mammograms, other radiologists did so half the time. For false positives, the range was 1–64 %. Another study[10] found that two psychiatrists who independently diagnosed 426 state hospital patients agreed only in half of the cases which mental illness the patient suffered from.
- Other types of costs: a meta-analysis[11] showed that a quarter of the time, two separate recruitment interviewers disagreed on which job candidate was the best fit for the job. This was despite the interviewers sitting on the same panel, thus having seen the candidates in the exact same circumstances. (The correlation between the recruiters’ judgments was 0.74.) In a study[1](pp28–29) at an insurance company, the median premiums set by underwriters independently for the same five fictive customers varied by 55 %. This which was about five times as much as expected by most underwriters and their executives. The authors point out that this was the median; half of the time the difference was even larger.
Types of noise[edit]
The following typology of the components of noise in human judgment has been proposed by Kahneman, Sibony and Sunstein in their 2021 book Noise: A Flaw in Human Judgment:[1]
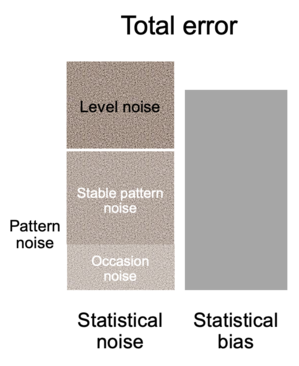
- Level noise, which arises due to differences between judges regarding how strict/careful/optimistic etc. they are in general. For example, some court judges tend to always issue harder sentences than others, no matter the crime.
- Stable pattern noise, arising because of permanent/semi-permanent differences between judges in how they react to or are able to deal with certain circumstances in what is being judged. For example, some court judges tend to always issue harder sentences than their colleagues do when the defendant has previous convictions.
- Occasion noise, which arises due to factors affecting judgment temporarily. For example, a court judge may have recently seen something on the news that has a connection to the judgment, such as a documentary on drug abuse, and this may affect how the judge views the case, and similar cases, during a period of time. Occasion noise can also arise due to factors that have no actual relation to the case, for example the judge's mood or level of energy/fatigue or stress.
An example of such occasion noise is the finding in a review study[12], looking at 207,000 immigration court decisions, that asylum applicants were less likely to be granted asylum on hot days. Other examples are studies finding that court judges were less lenient if they were hungry (when granting parole[13]) or if the local football team had just lost a game (both in general[14] and in juvenile court, especially for defendants of dark skin[15]).
Fig 2 illustrates the relationship between these components of noise, as well as how noise (unwanted variability) is one part of the total error in the judgments in question, with statistical bias (a shift in the average away from the true value) being the other.
As the figure shows, stable pattern noise and occasion noise together make out what is called pattern noise, which then can be described as noise that arises either because of differences between judges in how they interact with details in what is being judged, or because of transient factors affecting the judges differently. Level noise on the other hand reflects judges' beliefs regarding what the magnitude should be for the overall type of judgment in question.
Detecting noise[edit]
Noise is relatively hard to spot, and several possible explanations for why this might be the case have been proposed. These explanations include the following:[1]
- People do not want to believe that there could be so much unwanted variability between judges/judgments. The embarrassing nature of this fact may make us try to deny it, both to ourselves and to others.
- The human mind always want to blame a bias and therefore attempts to find meaning in randomness (see for example hindsight bias).
- People often think that other people reason as they do and hence may not suspect that there could be noise.
- People often consider bad decisions to be rare exceptions or outliers made by "bad apples", rather than being legitimate data points to consider.
- Detecting and measuring noise requires deliberate effort, which is not necessarily the case with bias. This is because noise is inherently statistical: it becomes visible only when we think statistically about an ensemble of similar judgments.[1](p204)
However, measuring noise has one advantage over measuring statistical bias: noise can be measured even when the true value of the judgment task is unknown (if there was no bullseye in Fig 1).[1]
A method that has been proposed for detecting noise is to perform a so-called noise audit.[1]
Reducing noise[edit]

Noise reduction techniques[edit]
Techniques to reduce noise include both relatively small changes to the choice architecture (the physical and psychological environment in which the judgment is made), such as nudges, and larger changes, with the most suitable type depending on the type of judgment in the situation.[1]
No matter the type used, the goal is to help judges be more uniform in their judgments – to decrease the range of judgments[1], as illustrated in Fig 3.
Noise reduction has also been called 'decision hygiene'.[1]
Noise reduction through using better judges[edit]
Noise reduction can be achieved by ensuring that the judges are good at the judgment task. This should reduce noise since if the judges are all skilled and all think about the problem/case etc. in consistent and suitable ways, their judgments should be similar to each other. Two ways to move in this direction are to select better judges and to train existing judges.[1]
Noise reduction through algorithms/hard rules[edit]
Noise reduction can be achieved by replacing human judgment with algorithms or hard rules, which is easier for certain types of decisions than others. It can be done for example for routine medical exams and for certain types of predictions, a domain in which well-designed algorithms routinely outperform human professional judges. It is harder for judgments such as in court trials, strategic business decisions and difficult medical diagnoses, since such judgments are made only rarely and typically in highly differing contexts.[1] Examples are algorithms for making fairer and more accurate bail decisions concerning flight risk[16] and to screen job applicants' résumés so as to increase the share of interviewed candidates that then are found suitable for the position[17]. Medical examples include the rules and procedures doctors use to quantify tendon degeneration, to evaluate core needle biopsies for detecting breast lesions and to diagnose strep throat.[1]
Noise reduction through helping human judgment[edit]
Noise reduction can be achieved by helping the judgment of existing judges. Some of the techniques for doing this can be used both in the same judgment tasks/domains as those suitable for hard rules and algorithms as well as in other areas, whereas some of the techniques are suitable mainly for more difficult decision problems. Since all of these techniques steer human judgment by guiding it through better, more psychologically informed processes, they are suitable for situations where judges or those judged express a need for human agency and human interaction, wishing not to be reduced to "non-thinking cogs in a machine".[1]
- Soft rules: With soft rules, judges receive help from rules/algorithms, at least in part of the judgment process. When soft rules are used during part of the process, they can decrease noise both in the stages they are explicitly used and in how judges then approach the remaining stages. Types of soft rules include guidelines, checklists and scoring rules. An example of a commonly used guideline across the world which decomposes a time-constrained judgment task into a multi-dimensional structured assessment is the Apgar score, which makes it easier for midwives and doctors to quickly judge the healthiness of newborns.[1]
- Debiasing: Here the aim is to hinder cognitive biases from creating noise. In other words to prevent faulty gut instinct from exercising undue influence in the judgment process. This can be done in different ways, but can turn out badly if a bias is assumed to be present but in fact is not.[1]
- Debiasing after the fact: For example adding a buffer/margin in order to be prepared if cost or time predictions turn out to be overoptimistic due to overconfidence bias.[1]
- Debiasing before the fact: For example to promote active open-mindedness, to give judges bias training, to use boosting (decision aids helping conscious judgment, broadly speaking), or to use nudging (small changes to the physical or psychological environment that can affect conscious or unconscious judgment).[1]
- Debiasing during the judgment: It has been proposed that it can be good to use well-chosen decision observers that are trained to spot – in real-time – biases affecting decisions. The rationale for this not least includes the bias blind spot, research that shows that people often recognize biases more easily in others than in themselves.[1]
- Impose a better process: This type of noise reduction has to do with favoring independence between different aspects of the judgment, promoting independence between individual judges, and with sequencing information in an appropriate way. Independence can mean both that different aspects of the judgment are assessed separately and that different judges are independent from each other, so as to not affect each other's judgment. Often, the aims are to delay holistic judgment until later, by structuring complex decisions and decomposing the judgment task into component sub-judgments. Only then, at the end, should all aspects be discussed/viewed at once, and it is often advisable to keep even that part of the process structured. An example application is when an executive team is to make a strategic business decision.[1]
- Put judgments in context: Rating things such as applicants, strategy prospects or employee performance on a numeric scale or similar often leads to noisy judgments. Ways to decrease this noise include to use rankings and better scales.[1]
- When ranking things, judges do not need to think hard about which rating to give. Instead, they can simply compare the thing in question to other, similar things. This makes the judgment task easier. However, ranking can backfire for example if judges are forced to distinguish between the indistinguishable or if an inappropriate scale is used (such as a relative scale used to measure absolute performance).[1]
- Using good scales can mean to use scales that either are standardized, are based on base rates (also called taking the outside view, which means to judge prospects based on how similar efforts have fared) or that use anchors such as descriptions of behaviors (as behaviorally anchored rating scales do) or vignettes (as case scales do).[1]
Noise reduction through aggregating human judgments[edit]

Noise reduction can also be achieved by aggregating many human judgments on the same problem/case etc, which is mathematically guaranteed noise reduction.One way to aggregate human judgments is to utilize the wisdom of crowds. This entails to aggregate judgments that are made independently from each other and preferably from diverse judges that complement each other. When the judgment is complex or expertise-specific, limiting the 'crowd' to selected experts is a good idea. A related but distinct judgment aggregation strategy is to utilize the wisdom of the inner crowd, also called the crowd within. This entails aggregating an individual judge's judgments on the same issue made on different occasions.[1]

Zero noise should not always be the goal[edit]
Kahneman, Sibony and Sunstein[1] have argued that although noise reduction most of the time is a worthy objective – depending of course on the context – zero noise should not always be the goal to strive for, not even in cases where noise can plausibly be reduced by a lot. First, reaching zero noise may not be feasible, for various reasons, not least due to costs and the fact that for many types of judgment some noise will always remain, because even though a lot can be done to rein in the peculiarities of the human brain, there is a limit to what can be accomplished. Second, reaching zero noise may not be desirable since it would reduce flexibility, especially if the noise reduction technique considered has to do with rules. In particular, this is because rules can be difficult to agree upon, update or design in a way that covers all potential eventualities. Sometimes standards are therefore a better way to go, even though doing so inevitably means more noise since standards are principles that rely on such noisy human processes as common sense and good faith.
Third, total noise reduction can stand in competition with other important values. One such value is to let judges have a sense of agency and feel fulfilment after having reached a decision, which can be important especially for judgments such as in business strategy or political forecasting or in other areas where professional pride plays a large part. Both the welfare of judges and effectiveness concerns come into play here, with effectiveness having to do with the fact that judges may rebel if they perceive that their ability to use their intuition and express their individuality and personal values is threatened. This for example happened with federal sentencing guidelines in the US, which led the guidelines to later be abandoned. Moreover, if judges lose these qualities, they often find a way to game the system and get the decision they want anyway. Fourth, another potentially competing value is the ability to harness important competencies and information that judges can possess that may go unutilized if there is too much noise reduction.[1]
For these reasons, there is often still room and need for intuition, consideration of particulars and discrete judgment – but not until noise reduction has been performed. The idea is to not rely on human judgment all the way, especially not snap judgment. Instead, carefully informed intuition that is less prone to error, thanks to noise reduction, should be used.[1]
Critique[edit]
Dehumanization[edit]
Loss of judges' professional pride
Critics of noise reduction have argued that noise reduction efforts can make judges feel like cogs in a machine, like little more than executers of mechanical solutions that embody an overly large technocratic faith in experts and central planning. Put differently, decreasing the space for noise to arise can increase rigidity, which may demoralize judges and squelch their creativity and sense of professional pride and agency.[3][1]
No human being on the other side of those judged
Critics of noise reduction have argued that people affected by the judgment made through a noise-reduced process can feel as if they are not treated as humans, because they have not had a human judge truly decide their case and have not been given an opportunity to influence the decision by talking to a human with the ability and mandate to influence or change the judgment. Put differently, those judged often wish to be treated with respect and dignity in individualized hearings.[1]
Ethics and other unintended consequences[edit]
Can introduce or shift bias
Critics of noise reduction have argued that such efforts risk leading to more bias, or other bias than what was present before, if the noise reduction technique misfires.[5][1] This could for example take the form of more racial bias or gender discrimination or that such or other biases replace the original biases. Such unintented consequences could for example arise if the noise reduction technique were to leave out important variables or include problematic ones. If this happens, the resulting situation would be biased not only sometimes but reliably so, thus magnifying human bias.[1]
Can focus dangerous bias
A related critique concerns the problem of asymmetric risk in judgments where there is both bias and noise. Noise reduction may be overly risky to perform when it comes to cases where the costs of error are asymmetrical.[5] For example, one such case would be a company that manufactures elevators. If the company miscalculates, the situation will be much worse if they overestimate the load the elevator can take than if they underestimate it. Other examples are military commanders deciding whether or not to launch an offensive and governments having to respond to a health crisis such as a pandemic.[1](p65) If a set of judgments is biased to the side of the real value which has asymmetrically large consequences, and noise reduction leads to lower variability in judgments, that means that a higher share of the judgments will be on the wrong side of the true value and thus a higher likelihood that catastrophes may occur, such as businesses making a faulty decision that ends up ruining the company. So noise reduction could be a bad idea for these judgments.[5] One reply to this critique is that noise reduction should ideally be followed by decision makers using the now-better judgment data together with their values and potential risk-avoidance criteria to make the optimal choice in the situation.[1](p65)
Sheer costs[edit]
Critics of noise reduction have argued that the costs of such efforts may be higher than the benefits. This argument has been met with admission that although there definitely are such cases, the opposite will more often hold: the costs of the noise are typically high and the costs of reducing it lower. Moreover, it has been said that one needs to think about noise reduction not only in terms of monetary costs: in some cases a noise reduction investment may not be recovered in dollars and cents (at least not in a way easily measured or in a way that directly benefits the organization in question), but the decreased injustice will nevertheless be well worth it.[1]
Common counter-reply[edit]
In Noise: A Flaw in Human Judgment, a central book on the concept of noise in human judgment, these critiques have all been replied to with the argument that they are fair mainly for some types of noise reduction techniques, such as guidelines, rules and algorithms. Even though these may however still be less problematic than depending on human judgment, especially since they are relatively easy to scrutinize and change, other noise reduction techniques can be applied instead. Doing so, it is said, can for example actually help judges use careful consideration and professional judgment better, can make judges more receptive to fresh ideas, can free up time that judges can spend on other, more complicated aspects, can give person being judged more individualized hearings with humans.[1]
See also[edit]
References[edit]
- ↑ 1.00 1.01 1.02 1.03 1.04 1.05 1.06 1.07 1.08 1.09 1.10 1.11 1.12 1.13 1.14 1.15 1.16 1.17 1.18 1.19 1.20 1.21 1.22 1.23 1.24 1.25 1.26 1.27 1.28 1.29 1.30 1.31 1.32 1.33 1.34 1.35 1.36 1.37 1.38 1.39 1.40 1.41 Kahneman, Daniel; Sibony, Olivier; Sunstein, Cass R. (2021). Noise: A Flaw in Human Judgment. New York: Little, Brown Spark. ISBN 978-0-00-830899-5. OCLC 1242782025. Search this book on
- ↑ 2.0 2.1 2.2 Kahneman, Daniel; Rosenfield, Andrew M.; Gandhi, Linnea; Blaser, Tom (2016-10-01). "Noise: How to Overcome the High, Hidden Cost of Inconsistent Decision Making". Harvard Business Review. ISSN 0017-8012. Retrieved 2021-07-09.
- ↑ 3.0 3.1 Donohoe, Paschal (2021-06-12). "Noise: A Flaw in Human Judgment review: penetrating study of decisions". The Irish Times. Retrieved 2021-07-19. Unknown parameter
|url-status=
ignored (help) - ↑ Criado Perez, Caroline (2021-06-03). "Noise by Daniel Kahneman, Olivier Sibony and Cass Sunstein review – the price of poor judgment". The Guardian. Retrieved 2021-07-16. Unknown parameter
|url-status=
ignored (help) - ↑ 5.0 5.1 5.2 5.3 Blastland, Michael (2021-06-24). "Signal failure: Daniel Kahneman's fascinating—and flawed—new book 'Noise'". Prospect Magazine. Retrieved 2021-07-19. Unknown parameter
|url-status=
ignored (help) - ↑ Clancy, Kevine; Bartolomeo, John; Richardson, David; Wellford, Charles (1981-01-01). "Sentence Decisionmaking: The Logic of Sentence Decisions and the Extent and Sources of Sentence Disparity". Journal of Criminal Law and Criminology. 72 (2): 524–554. doi:10.2307/1143005. JSTOR 1143005.
- ↑ Simonsohn, Uri (2007). "Clouds make nerds look good: field evidence of the impact of incidental factors on decision making". Journal of Behavioral Decision Making. 20 (2): 143–152. doi:10.1002/bdm.545. ISSN 1099-0771.
- ↑ Chen, Daniel L.; Philippe, Arnaud (2020-01-24). "Clash of Norms: Judicial Leniency on Defendant Birthdays". SSRN Electronic Journal: 27 pp. SSRN 3203624.
- ↑ Beam, Craig A.; Layde, Peter M.; Sullivan, Daniel C. (1996-01-22). "Variability in the Interpretation of Screening Mammograms by US Radiologists: Findings From a National Sample". Archives of Internal Medicine. 156 (2): 209–213. doi:10.1001/archinte.1996.00440020119016. ISSN 0003-9926. PMID 8546556.
- ↑ Aboraya, Ahmed; Rankin, Eric; France, Cheryl; El-Missiry, Ahmed; John, Collin (2006). "The Reliability of Psychiatric Diagnosis Revisited: The Clinician's Guide to Improve the Reliability of Psychiatric Diagnosis". Psychiatry (Edgmont). 3 (1): 41–50. ISSN 1550-5952. PMC 2990547. PMID 21103149.
- ↑ Huffcutt, Allen I.; Culbertson, Satoris S.; Weyhrauch, William S. (2013). "Employment Interview Reliability: New meta-analytic estimates by structure and format". International Journal of Selection and Assessment. 21 (3): 264–276. doi:10.1111/ijsa.12036. ISSN 1468-2389. Unknown parameter
|s2cid=
ignored (help) - ↑ Ramji-Nogales, Jaya; Schoenholtz, Andrew I.; Schrag, Philip G. (2007). "Refugee Roulette: Disparities in Asylum Adjudication". Stanford Law Review. 60 (2): 295–411. ISSN 0038-9765. JSTOR 40040412.
- ↑ Danziger, Shai; Levav, Jonathan; Avnaim-Pesso, Liora (2011-04-26). "Extraneous factors in judicial decisions". Proceedings of the National Academy of Sciences. 108 (17): 6889–6892. Bibcode:2011PNAS..108.6889D. doi:10.1073/pnas.1018033108. PMC 3084045. PMID 21482790.
- ↑ Chen, Daniel L.; Loecher, Markus (2019-09-21). "Mood and the Malleability of Moral Reasoning". SSRN Electronic Journal: 62 pp. SSRN 2740485.
- ↑ Eren, Ozkan; Mocan, Naci (2018). "Emotional Judges and Unlucky Juveniles". American Economic Journal: Applied Economics. 10 (3): 171–205. doi:10.1257/app.20160390. ISSN 1945-7782. Unknown parameter
|s2cid=
ignored (help) - ↑ Jung, Jongbin; Concannon, Connor; Shroff, Ravi; Goel, Sharad; Goldstein, Daniel G. (2020). "Simple rules to guide expert classifications". Journal of the Royal Statistical Society, Series A (Statistics in Society). 183 (3): 771–800. doi:10.1111/rssa.12576. ISSN 1467-985X. Unknown parameter
|s2cid=
ignored (help) - ↑ Cowgill, Bo (2018). "Bias and Productivity in Humans and Algorithms: Theory and Evidence from Résumé Screening". Paper presented at Smith Entrepreneurship Research Conference, College Park, MD, April 21, 2018. Retrieved 2021-07-13. Unknown parameter
|url-status=
ignored (help); Unknown parameter|s2cid=
ignored (help) - ↑ Surowiecki, James (2004). The wisdom of crowds: why the many are smarter than the few. Doubleday; Anchor. ISBN 978-0-349-11605-1. OCLC 1040967876. Search this book on
This article "Noise (human judgment)" is from Wikipedia. The list of its authors can be seen in its historical and/or the page Edithistory:Noise (human judgment). Articles copied from Draft Namespace on Wikipedia could be seen on the Draft Namespace of Wikipedia and not main one.